If you’re developing AI models in Python but need to integrate them into a .NET application, here are some effective ways to bridge the gap:
1️⃣ Expose Python AI Models as a Web API (Best Approach)
✅ Best For: Scalable web apps, microservices, cloud-based AI
🔹 How it Works:
- Use FastAPI or Flask in Python to expose the AI model as a REST API.
- Consume the API in your .NET app using HttpClient in C#.
- Deploy the API as a Docker container, Azure Function, or AWS Lambda for scalability.
🔧 Steps:
In Python (FastAPI Example)
from fastapi import FastAPI
import joblib
import numpy as np
app = FastAPI()
model = joblib.load("model.pkl") # Load trained model
@app.post("/predict/")
def predict(data: dict):
input_data = np.array(data["features"]).reshape(1, -1)
prediction = model.predict(input_data)
return {"prediction": prediction.tolist()}
In .NET (C#)
using System.Net.Http;
using System.Text.Json;
using System.Threading.Tasks;
public async Task<string> GetPredictionAsync(double[] features)
{
using var client = new HttpClient();
var requestBody = new StringContent(JsonSerializer.Serialize(new { features }), Encoding.UTF8, "application/json");
var response = await client.PostAsync("http://localhost:8000/predict/", requestBody);
return await response.Content.ReadAsStringAsync();
}
🚀 Pros
✔ Scalable & cloud-ready
✔ Works well with microservices
✔ Can be hosted on Azure, AWS, or Docker
❌ Requires managing a separate Python API
2️⃣ Use Python.NET to Directly Call Python from C#
✅ Best For: Calling Python functions from C# without an API
🔹 How it Works:
- Use Python.NET (
pythonnet
library) to execute Python scripts within a C# app. - Great for local AI inference but not ideal for production-scale apps.
🔧 Steps:
Install Python.NET in C#
pip install pythonnet
using Python.Runtime;
public class AIModel
{
public void RunPythonModel()
{
using (Py.GIL()) // Acquire Global Interpreter Lock
{
dynamic np = Py.Import("numpy");
dynamic model = Py.Import("my_model");
var inputArray = np.array(new double[] {1.2, 3.4, 5.6});
var result = model.predict(inputArray);
Console.WriteLine($"Prediction: {result}");
}
}
}
🚀 Pros
✔ No need for a separate API
✔ Direct Python-C# interaction
❌ Requires Python installed on the server
❌ Slower than compiled .NET libraries
3️⃣ Run Python Scripts Using Process Execution
✅ Best For: Simple, lightweight AI integrations
🔹 How it Works:
- Execute a Python script from C# using
Process.Start()
. - Pass input/output via command-line arguments or files.
- Works well for batch processing but not for real-time AI.
🔧 C# Code
using System.Diagnostics;
public class PythonAI
{
public static string RunPythonScript(string inputData)
{
ProcessStartInfo start = new ProcessStartInfo();
start.FileName = "python";
start.Arguments = $"ai_script.py \"{inputData}\"";
start.RedirectStandardOutput = true;
start.UseShellExecute = false;
start.CreateNoWindow = true;
using (Process process = Process.Start(start))
{
using (StreamReader reader = process.StandardOutput)
{
return reader.ReadToEnd();
}
}
}
}
🚀 Pros
✔ Simple & easy to implement
✔ Works on Windows/Linux servers
❌ Slower due to process overhead
❌ Harder to manage in large-scale apps
4️⃣ Convert AI Models to ONNX for Native .NET Execution
✅ Best For: Running AI models inside .NET apps without Python
🔹 How it Works:
- Convert a trained model to ONNX (Open Neural Network Exchange) format.
- Use ONNX Runtime in .NET to execute the model efficiently.
🔧 Convert AI Model to ONNX in Python
import torch
import torch.onnx
model = torch.load("model.pth") # Load trained PyTorch model
dummy_input = torch.randn(1, 3, 224, 224) # Example input
torch.onnx.export(model, dummy_input, "model.onnx")
🔧 Use ONNX Model in C#
using Microsoft.ML.OnnxRuntime;
using Microsoft.ML.OnnxRuntime.Tensors;
var session = new InferenceSession("model.onnx");
var inputTensor = new DenseTensor<float>(new float[] {1.2f, 3.4f, 5.6f}, new[] { 1, 3 });
var inputs = new List<NamedOnnxValue> { NamedOnnxValue.CreateFromTensor("input", inputTensor) };
var results = session.Run(inputs);
🚀 Pros
✔ Faster inference (C# runs the model natively)
✔ No Python dependency
❌ Requires converting the model to ONNX
❌ Not all models convert cleanly
Which Approach Should You Choose?
Use Case | Best Approach |
---|---|
Web-based AI services | Expose Python AI model as a REST API (FastAPI/Flask) |
Direct Python usage inside .NET | Python.NET |
Simple AI execution | Process execution (Process.Start() ) |
High-performance, native .NET AI | Convert model to ONNX & use ONNX Runtime |
Final Thoughts
For most real-world applications, exposing Python AI models via a REST API is the best approach because it’s scalable, maintainable, and cloud-friendly. If performance is a priority, converting to ONNX can bring the best of both worlds.
💡 Which approach suits your project best? Let me know in comments, and I can help fine-tune the implementation! 🚀
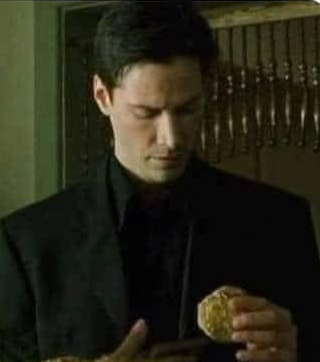
Amit Mittal is a seasoned tech entrepreneur and software developer specializing in SaaS, web hosting, and cloud solutions. As the founder of Bitss Techniques, he brings over 15 years of experience in delivering robust digital solutions, including CMS development, custom plugins, and enterprise-grade services for the education and e-commerce sectors.